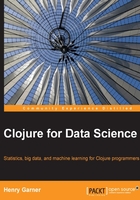
The central limit theorem
We encountered the central limit theorem in the previous chapter when we took samples from a uniform distribution and averaged them. In fact, the central limit theorem works for any distribution of values, provided the distribution has a finite standard deviation.
It doesn't matter that the underlying distribution is exponential—the central limit theorem shows that the mean of random samples taken from any distribution will closely approximate a normal distribution. Let's plot a normal curve over our histogram to see how closely it matches.
To plot a normal curve over our histogram, we have to plot our histogram as a density histogram. This plots the proportion of all the points that have been put in each bucket rather than the frequency. We can then overlay the normal probability density with the same mean and standard deviation:
(defn ex-2-7 [] (let [means (->> (load-data "dwell-times.tsv") (daily-mean-dwell-times) (i/$ :dwell-time)) mean (s/mean means) sd (s/sd means) pdf (fn [x] (s/pdf-normal x :mean mean :sd sd))] (-> (c/histogram means :x-label "Daily mean dwell time (s)" :nbins 20 :density true) (c/add-function pdf 80 100) (i/view))))
This code generates the following chart:

The normal curve plotted over the histogram has a standard deviation of approximately 3.7 seconds. In other words, this quantifies the variation of each daily mean being relative to the grand mean of 90 seconds. We can think of each day's mean as a sample from the overall population with the earlier curve representing the distribution of the sample means. Because 3.7 seconds is the amount that the sample's mean differs from the grand mean, it's referred to as the standard error.